Traditionally, validating massive volumes of financial data relied on static, rules-based approaches that attempt to flag anomalous data. These processes tend to be operationally inefficient, generating significant false positives while potentially failing to uncover particular errors. In this Q&A with Jeff Shortis, State Street Alpha Data Platform Product Owner, we discuss how State Street is harnessing AI to transform and improve data validation for investment firms and asset owners.
Jeff: It all starts with having access to consistent, high quality data for analysis. Firms need to ingest, validate, aggregate and distribute massive volumes of data to AI modeling engines. If there are flaws in the data or it’s not properly synchronized with related data elements, then the AI outputs derived from that data aren’t going to be accurate or useful.
Secondly, AI tools are sophisticated, computationally intensive technologies. They require on-demand scalability and elasticity, so having both your data and AI environment in the cloud streamlines workflows and significantly lowers operational overhead. Hence, firms need to make investments in the types of resources that can optimize their usage of cloud technologies. That requires both specialized talent to configure and manage these environments and individuals with expertise in AI, financial services and specific data domains, including multiple structured and unstructured data sources across both public and private markets.
To summarize, firms looking to launch best-in-breed AI initiatives need high quality data, a cloud-native environment, and the expertise to manage that environment and bring AI-based solutions and services to market for their stakeholders.
Jeff: Errors have ripple effects. Data rework is the most obvious cost – problematic data has been detected and the data ops teams need to diagnose the source of the issue, fix the error and propagate the corrected data to downstream systems and stakeholders. This often also involves interacting with external data vendors. Depending on the time elapsed since the error was detected, published information will need to be retracted and correction notices issued.
If that data was used in some critical process and decisions were made based on incorrect data, the firm may incur liability-related costs from their clients or investors. In the case of pensions and superannuation funds, this could conceivably impact the client’s beneficiaries. While hard to quantify, reputational costs can be considerable, resulting in client terminations.
Even if an error is caught early, the diagnosis and rework can result in delayed decision making, resulting in potential lost investment opportunities. Finally, depending on the magnitude of the data error, the firm may be subject to regulatory fines.
Jeff: The AI potential is enormous for firms with the breadth and depth of data required to arrive at a particular business insight. At State Street, our Alpha Data Platform serves as the foundation for AI initiatives, capturing and curating data across the client’s front, middle and back office, and enriching it with third-party analytics, benchmark, pricing and index data.
It’s critical that the data is consistent and accurate, so deploying AI for data validation and anomaly detection became one of our first important use cases. If you consider the history of data quality, going back centuries when somebody compared a value from a bank ledger to a value in an account, it’s been a manual, paper-based process. Not much changed until relational databases were launched in the 1970s. Data operations teams were able to validate data in a more efficient and scalable way using a rules-based approach to determine whether data elements exceeded pre-defined thresholds.
Soon after, spreadsheets became commercially available, enabling nontechnical users to do these sorts of analyses. A cottage industry of data quality vendors has cropped up, but they're simply using threshold-based techniques developed decades ago to automate data quality assessments. That still requires significant human expertise to establish appropriate thresholds and identify true anomalies in their data. For example, if I know that a bond coupon should be calculated in a particular way, or if a country code can only be in a particular list of valid values, or if prices need to be within a certain tolerance, I write rules to encapsulate that logic. This is a reactionary approach that can generate lots of false positives while potentially missing actual errors that just happen to pass the validation rule.
AI comes at this from a completely different direction. It’s proactive and doesn’t depend on writing hundreds of rules to determine whether there's a problem or not. The AI model “learns” what’s normal and what's suspect, and flags exceptions for further investigation, allowing anomalies to be identified much sooner. This prevents the ripple effect of downstream problems and significantly reduces the amount of rework required. AI offers the additional benefit of being applicable across any data domain, from pricing to reference data, to transactions, index and margin data.
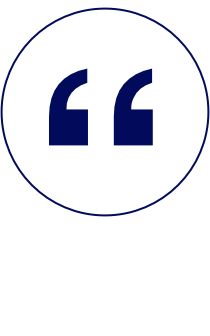
You need to apply AI technology so that you can then subsequently feed it into other technologies. And that is what we've done, creating domain-specific AI models which are able to understand data structure and manage the provenance of your data to deliver consistently high-quality data.
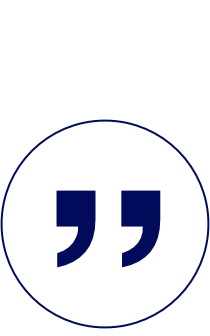
We created this feedback loop with our operations teams, wherein we are able to remove false alerts, while continuously learning and evolving what qualifies as a true positive. Our models keep learning from new data, becoming increasingly more efficient. By applying AI and removing thousands of false alerts, we have made our operations teams 25 times more efficient.
In terms of the ability to address, identify and resolve data quality issues, I think AI is the most important breakthrough since the invention of the database. We've been working on these capabilities for over two years, leveraging our research-oriented data science and engineering teams. These capabilities are in production today, providing our clients with value across their investment and operations teams.
Jeff: I think it's crucial that they do so. First, the massive investment that it takes to effectively extract value from these initiatives we discussed earlier requires assembly of large, complex and high-quality data sets. Having the expertise to leverage cloud native capabilities and access to subject matter experts and resources to stand up AI oriented analysis requires a huge investment.
State Street has been doing this for years and we have teams in place globally that allow us to accelerate AI initiatives. Secondly, we have strategic relationships with vendors like Microsoft Azure and Snowflake, with the ability to help shape their product roadmap. Working with a firm that has the controls, depth of knowledge and breath of financial services experience are a must when selecting a strategic partner.
At State Street, we've established both internal and external steering committees. Our internal subject matter experts understand these use cases from the front-to-back perspective. We also leverage our client’s expertise to triangulate, prioritize and shape those use cases. Clients can benefit from the relationships and teams we’ve built as a means of advancing their growth.
Learn more about what State Street Alpha can do for you.
Please provide your information and a member of our client services team will contact you promptly.